Why is coming to common metric for measuring influence so hard? Short answer: because measuring influence is not only nuanced, but it’s also really hard. Maybe we’re asking the wrong question, maybe we should be asking how susceptible to influence are we?
First, a matter of semantics: authority is power bestowed by an outside source. Police, judges, your boss, etc. all have power over you in their own contexts. In most cases, authority is external to Social Media, so what we really want to know is how influential (power regardless of authority) a person is. So, I’ll stop talking about authority and start talking about influence.
Influence in SM is created through exertion of control over content that reaches you by modifying the content, or adding additional context such as your opinion. Modifications can be explicit, or implicit; merely passing along a piece of information indicates you have some interest in it. The social part of this information flow dictates who sees your content. So your influence is relative to your network. That’s bad for good metrics. What worse, from the following diagram, you can start to see that influence is also relative to the individuals within your network. Fortunately, combining influence and reach seems to be promising.
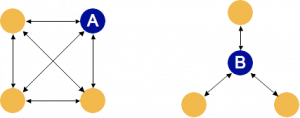
Imagine you are A. You’re going to have a lot easier harder time exerting control over information flowing through your network than B. That’s an example, why reach (or count) alone is a poor measure of influence. But, clearly if you have a larger network, you are likely to influence more people. So how do quantity and influence work together? One strong way is path independence.
Turning influence on its head, it is much easier to measure how likely we are to be influenced depending on where the content comes from. Hearing from two independent sources will have greater impact on our decision forming than two related sources. In marketing, this is called the media multiplier when advertising is spread across multiple channels, e.g. Radio + TV.
So maybe the real question is, how influencable are we? One easy measure is network efficiency.